The power of Generative AI for your business
We can't deny it: 2023 was a groundbreaking year in which Generative AI tools became increasingly entrenched in society and business. However, we are still often asked how Generative AI works and how it differs from “normal” AI, a buzzword that has been around for several decades now. In this blog, we explain in detail how this technology works, how it relates to Artificial Intelligence and where the opportunities lie for your company. And that in a technically substantive but understandable way.
.jpg)
What is Generative AI?
First things first: to thoroughly understand how Generative AI works, we'll take a look at the basic definition. Generative AI is a technology that allows you to generate new content, such as texts, audio clips, videos, images, or code. It learns from large amounts of data and then generates different types of output. ChatGPT (text), Midjourney (images) and Microsoft Copilot (Office suite support) are among the best-known Generative AI tools of the moment. Note: Generative AI is only a fraction of the bigger picture.
AI, Machine Learning, Deep Learning, and Generative AI
Artificial Intelligence (AI) is an overarching concept and includes various technologies. Specifically, it refers to machines that mimic capabilities that actually require human intelligence.
One of those exciting technologies is Machine Learning (ML). It focuses on one specific task and performs it very intelligently. We feed the Machine Learning Model with data and rules, allowing it to perform analyses and predictions independently. Some examples include voice and image recognition and recommendation systems such as Netflix, which makes suggestions based on your viewing habits. The main limitation of Machine Learning, however, is that it requires human interaction when adding new data sets to correct errors.
That is exactly why Deep Learning (DL) was later created as a method to modify results using a proprietary neural network, without human intervention, to improve the accuracy of the output. We'll explain exactly how these neural networks work later in this blog. In addition, Deep Learning works with millions of neurons or data points, which is far more than with Machine Learning technology. Self-driving cars are an example of Deep Learning. After all, they use neural networks for object recognition or pedestrian tracking to navigate their surroundings.
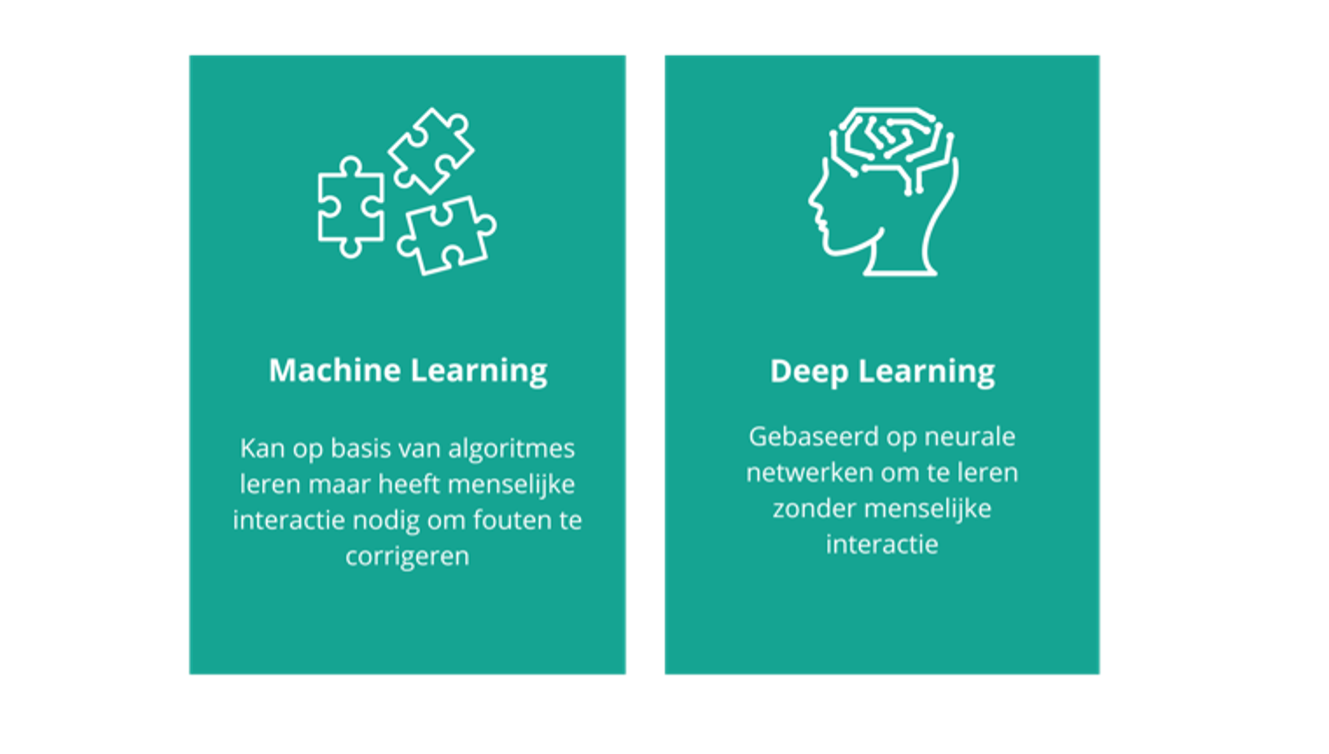
Based on the Deep Learning technique and the millions of data points, Generative AI was eventually created. This technology is capable of generating completely new content based on historical data.
In short, Machine Learning recognizes patterns and tells what it has learned from data sets, Deep Learning does this with huge amounts of data without human interaction, and Generative AI also produces completely new content.
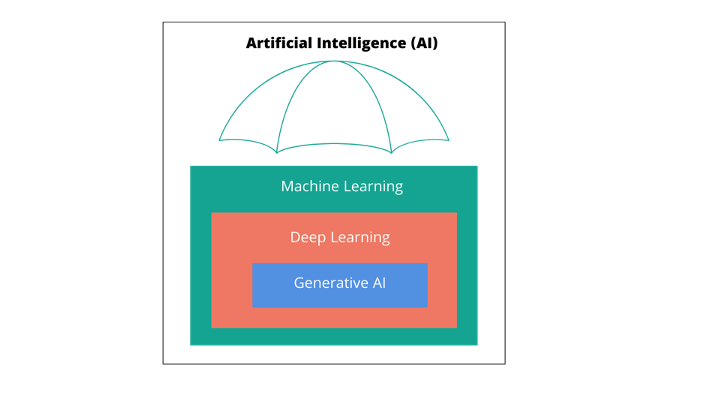
Generative AI: Neural Networks
Now that we understand how the different AI technologies relate to each other, let's look at how Generative AI works. To do that, we first need to understand what neural networks are and how they work.
Neural networks are based on the functioning of neurons in our brain, where countless neurons are interconnected. Each neuron responds with a certain intensity — strong or weak — to a stimulus it receives. By connecting different neurons, we eventually arrive at a certain outcome, such as distinguishing different letters.
In fact, a neural network is a complex computation of input, processing, and output. This processing takes place via one or more layers of neurons.
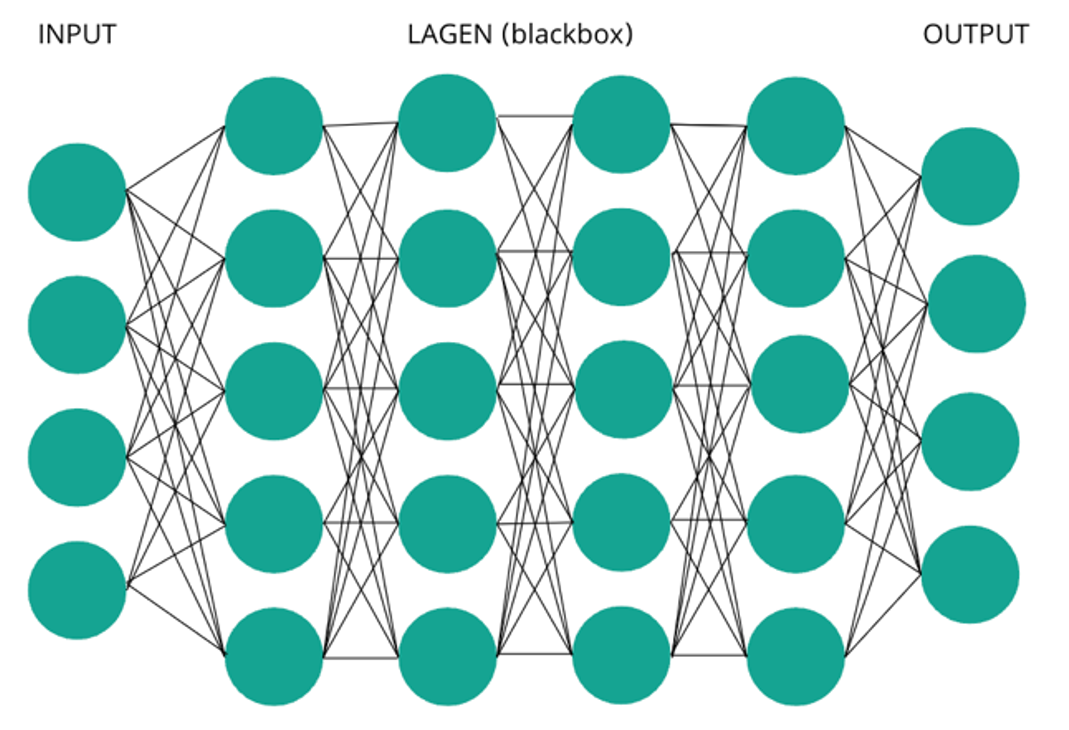
To clarify the somewhat abstract theory behind neural networks, we would like to illustrate it with an example: facial recognition by your smartphone. The neural network is trained to recognize the unique features of your face, such as the distance between your eyes and the shape of your nose. This happens because it transmits pixels to the input layer. It then rates each pixel individually with a score of 0 to 1, which indicates how much it matches a specific pixel in your face. By sending thousands of pixels through thousands of layers, the model identifies a face. These weightings can also be adjusted to make the model more accurate through further training.
Foundation Models and Generative AI
Foundation Models form the core of Generative AI and are trained on massive amounts of generic data. They perform general tasks such as understanding language, generating texts and images, and even communicating via language. These models form the basis for Generative AI and are also essential for producing new content.
An example of such a Foundation Model is a language model, or a large language model, that is trained on various text sources that you can find on the internet. Books, websites, blogs, and forums are just a few examples. OpenAI's well-known ChatGPT is one such large language model. This model statistically learns which word often follows another word in a given language, allowing it to generate complete and coherent sentences.
Transformers and vectors
Let's go one step further to really understand the basics of Generative AI. Generative AI is based on a new neural network architecture invented in 2017. This technology transforms words into vectors, or lists of numbers, in order to obtain a mathematical representation of a word.
A vector is placed on a specific dimension in a matrix to include both the meaning and context of a word and to show how relatively close a word is to another word.
In cases like “it's hot in summer” and “it's cold in winter”, “warm” and “summer” and “cold” and “winter” are close together on the “temperature in a season” dimension, while “summer” and “winter” are close together on the “seasons” dimension. In this way, the model is able to form accurate sentences with words that are statistically common in each other's neighborhood.
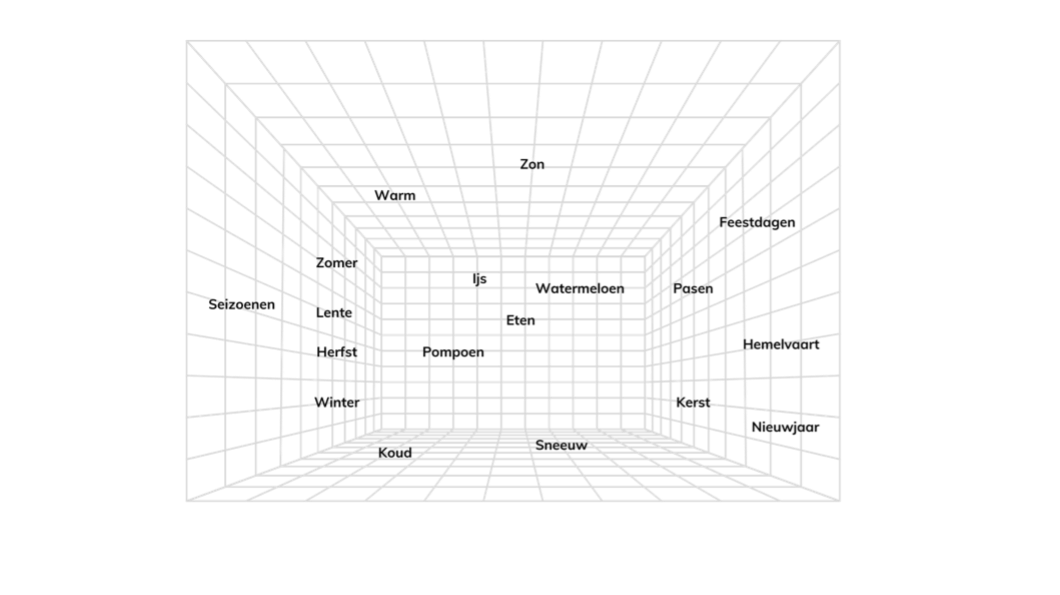
Why the rapid evolution?
The technology around neural networks and machine learning has been around since the 1960s, but back then, computing power was not powerful enough and there was no sufficient available data to train models. That has changed enormously over the past decade, so we are now seeing rapid progress in the AI landscape. Moreover, the architecture on which Generative AI is based has only recently been developed, and it is already clear that this offers many new possibilities.
What about my privacy?
One of the most common questions we get is about privacy and the reluctance to share our own business information. Sharing business documents with large language models, such as OpenAI's ChatGPT, is not a good idea. After all, this data could be used to improve their own model. However, there is no need to panic. After all, these Generative AI tools offer licensing models that guarantee data privacy and security. For example, OpenAI has a private environment, specifically for companies, where the data is protected and not externally accessible. Other major players, such as Google, Microsoft and Amazon, also offer private servers via their cloud platforms, where third parties do not have access to your data, just like when using their cloud storage to store documents.
Do you have doubts, right? Then it is best to check the company policy. These usually indicate what is or is not allowed when it comes to exchanging data. As a business manager, it is best to be informed about this by specialized organizations with expertise in the field of regulations and the opportunities for your company.
What's next?
Generative AI opens doors to countless new possibilities that were previously unthinkable, such as providing insight into unstructured data, including information hidden in Word or Excel files, PowerPoints, PDFs, emails, and chat messages that is not directly stored in a database, CRM or ERP system. Thanks to Generative AI and the rise of Foundation Models, it is now possible to interpret this data and make it transparent.
There are many options for companies to use Generative AI as part of their business processes. Some examples of use cases include...
• Generating quotes based on previous offers
• Summarizing and analyzing a specification and then assessing whether this specification offers added value for your company
• Approaching software in a different way, for example by creating dashboards based on chat prompts such as “give me a margin comparison for our top 10 customers between quarter 1 of last year and this year”
• Setting up your own company GPT to make company policies accessible to staff, for example “what should I do if I have an accident with my company car?”
• Sending personalized emails to customers, based on that customer's CRM data
However, this technology is still in its early stages and is considered to be the biggest technological revolution since the rise of the Internet and mobile phones. While 2023 was mainly about experimenting and developing proof of concepts, in 2024 we will mainly see an adoption of this technology, stimulated by the launch of new opportunities within existing business software. In addition, we experience a lot of interest and traction from companies that want to deal hands-on with these new opportunities in their search for a competitive advantage.
Do you want to gain a competitive advantage yourself and use Generative AI as part of your business processes, but you don't know where to start? Ask us anything! Quickly schedule a free intake interview, and we'll look at the options together.